New paper: Generative Adversarial Networks in the built environment: A comprehensive review of the application of GANs across data types and scales
Building and Environment publishes our new review paper.
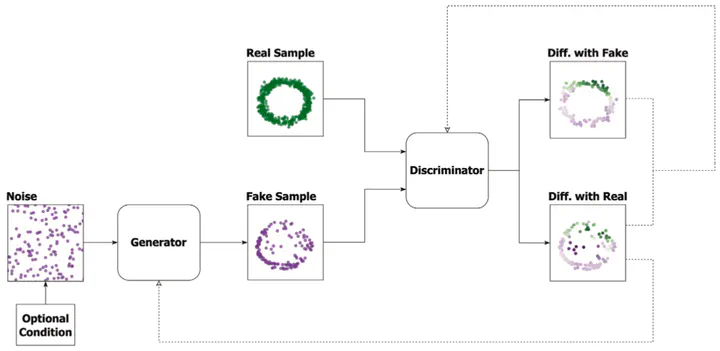
We are glad to share our new paper:
Wu AN, Stouffs R, Biljecki F (2022): Generative Adversarial Networks in the Built Environment: A Comprehensive Review of the Application of GANs across Data Types and Scales. Building and Environment 223: 109477. 10.1016/j.buildenv.2022.109477 PDF
This review paper was led by Abraham Noah Wu. Congratulations on his continued publication successes into his academic career. 🙌 👏
Abstract
The abstract follows.
Generative Adversarial Networks (GANs) are a type of deep neural network that have achieved many state-of-the-art results for generative tasks. GANs can be useful in the built environment, from processing large-scale urban mobility data and remote sensing images at the regional level, to performance analysis and design generation at the building level. We analyzed 100 articles to provide a comprehensive state-of-the-art review on how GANs are currently applied to solve challenging tasks in the built environment. Our results show that: (i) GANs are replacing older methods in some problems and setting state-of-the-art performances; (ii) GANs are opening new frontiers in previously overlooked problems, such as automatically generating spatially accurate floorplan layouts; (iii) GANs can be applied to different scales in the built environment, from entire cities to neighborhoods and buildings; and (iv) GANs are being used in a variety of problems and data types, from remote sensing data augmentation, vector data generation, spatio-temporal data privacy protection, to building design generation. In total, there are 26 unique application domains enabled by GANs; (v) however, one common challenge in this field currently is the lack of high-quality datasets curated specifically for problems in the built environment. With more data in the future, GANs could potentially produce even better results than today.
Paper
For more information, please see the paper, published open access.
BibTeX citation:
@article{2022_bae_gan,
author = {Wu, Abraham Noah and Stouffs, Rudi and Biljecki, Filip},
journal = {Building and Environment},
title = {Generative Adversarial Networks in the Built Environment: A Comprehensive Review of the Application of GANs across Data Types and Scales},
year = {2022},
pages = {109477},
volume = {223},
doi = {10.1016/j.buildenv.2022.109477}
}